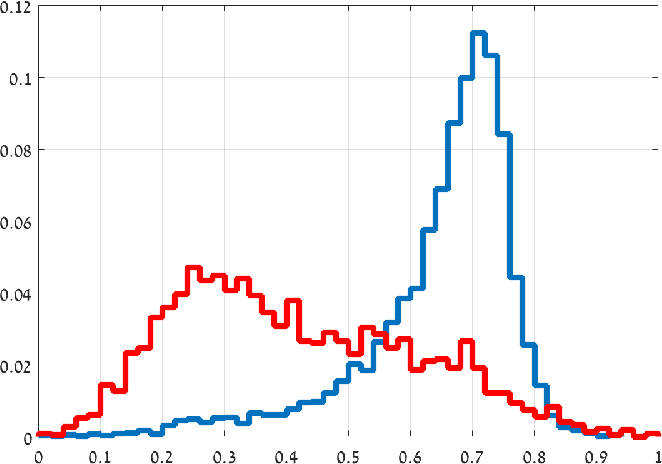
Figure 2 From Baby Cry Detection In Domestic Environment о Abstract and figures. automatic detection of a baby cry in audio signals is an essential step in applications such as remote baby monitoring. it is also important for researchers, who study the. Automatic detection of a baby cry in audio signals is an essential step in applications such as remote baby monitoring. it is also important for researchers, who study the relation between baby cry patterns and various health or developmental parameters. in this paper, we propose two machine learning algorithms for automatic detection of baby cry in audio recordings. the first algorithm is a.
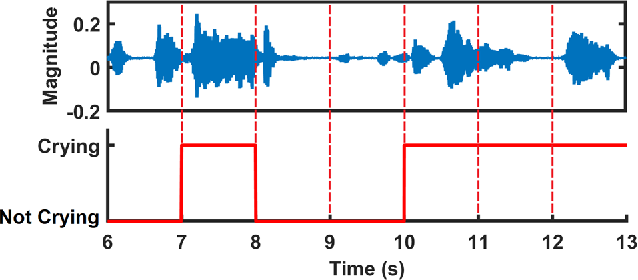
Figure 2 From Real Time Baby Crying Detection In The Noisy Everyday Segment age. detection of baby cry signals is essential for the pre processing of various applications involving crial analysis for baby caregivers, such as emotion detection. since cry signals hold baby well being information and can be understood to an extent by experienced parents and experts. we train and validate the. A baby cry is elicited from rhythmical transitions between inhalation and exhalation, due to a vibration of the vocal cords that produces periodic air pulses. the period of these pulses is called the fundamental frequency (pitch), and its typical values in healthy babies are 250 − 600 hz. In this chapter, we compare deep learning and classical approaches for detection of baby cry sounds in various domestic environments under challenging signal to noise ratio conditions. Recently, deep learning approaches have been considered to detect cries in a domestic environment (lavner et al 2016, torres et al 2017 or the nicu (ferretti et al 2018). in all studies, log mel.
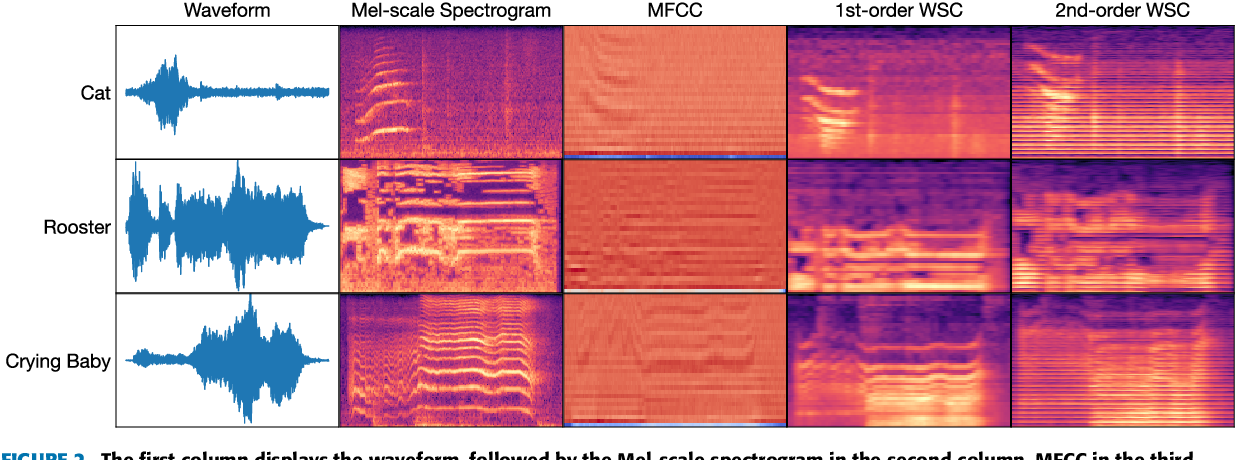
Figure 2 From Infant Cry Detection With Lightweight Wavelet Scattering In this chapter, we compare deep learning and classical approaches for detection of baby cry sounds in various domestic environments under challenging signal to noise ratio conditions. Recently, deep learning approaches have been considered to detect cries in a domestic environment (lavner et al 2016, torres et al 2017 or the nicu (ferretti et al 2018). in all studies, log mel. 2020. tldr. a real time baby crying detection algorithm that monitors the noisy environment for baby crying on a second by second basis is proposed and the designed algorithm detected baby crying through five acoustic features – average frequency, pitch frequency, short time energy acceleration, zero crossing rate (zcr), and mel frequency. Performance evaluation of the algorithms is carried out using an annotated database containing recordings of babies (0 6 months old) in domestic environments. in addition to baby cry, these recordings contain various types of domestic sounds, such as parents talking and door opening.

Baby Cry Detection In Domestic Environment Using Deep Learning 2020. tldr. a real time baby crying detection algorithm that monitors the noisy environment for baby crying on a second by second basis is proposed and the designed algorithm detected baby crying through five acoustic features – average frequency, pitch frequency, short time energy acceleration, zero crossing rate (zcr), and mel frequency. Performance evaluation of the algorithms is carried out using an annotated database containing recordings of babies (0 6 months old) in domestic environments. in addition to baby cry, these recordings contain various types of domestic sounds, such as parents talking and door opening.
Comments are closed.